As services and industries accept AI-driven services, one programming language has actually become the giant driving this transformation – Python. From reinventing data scientific research to crafting intelligent systems, Python has solidified its position as the best language for ML fanatics and specialists, highlighting its duty in the realm of Python for machine learning.
Python’s flexibility equips developers to create innovative ML designs that satisfy diverse needs and sectors, from responsive equipment to independent AI. Join us as we untangle the keys to Python’s supremacy in ML and find how this amazing language remains to shape the future of AI-driven developments.
- Python’s versatility, readable syntax, and extensive libraries make it a powerful choice for ML.
- Python supports various levels of AI sophistication, from reactive machines to those with self-awareness.
- Python excels in ML due to its simplicity, abundant libraries, platform independence, and active community support.
The Rise of Machine Learning in Big Business
Machine learning is transforming how industries operate, pushing the boundaries of what businesses can achieve. In sectors like healthcare, finance, automotive, and retail, machine learning is not only boosting efficiency but also unlocking new opportunities. For instance, in healthcare, machine learning algorithms are used to predict patient outcomes, streamline diagnostics, and assist with personalized treatment plans. In finance, Python for machine learning models is employed to detect fraudulent activities, forecast market trends, and automate trading processes. These advancements highlight the power of machine learning in driving innovation and maintaining a competitive edge.
Large enterprises have already seen significant benefits from integrating Python for machine learning into their operations. E-commerce giants utilize machine learning to refine their recommendation engines, leading to increased sales and customer satisfaction. Automotive companies harness Python for the advancement of autonomous vehicle technology, enhancing safety and efficiency. In the banking sector, Python-powered machine learning models are crucial for credit scoring and risk management, offering more accurate assessments and better decision-making. These examples illustrate how Python for machine learning is driving growth and operational excellence in large businesses.
How does Python work?
Many individuals currently choose Python as their preferred language for Artificial intelligence (ML) and Artificial Intelligence (AI) applications because it is functional and uncomplicated. Understanding how Python operates sheds light on its prevalence in data scientific research and ML, showcasing its significance in the realm of Python for machine learning.
- Readable and Intuitive Syntax
Python’s design belief system prioritizes code meaningfulness, making it justifiable and kept up. Its clean and brief language structure shows up like a common language, making it conceivable for creators to precise complicated thoughts expeditiously.
- Interpreted and High-Level Nature
As a translated language, Python does not call for compilation, enabling real-time execution and debugging.
- The abundance of Libraries and Frameworks
Python’s strong environment of ML collections and structures, such as TensorFlow, PyTorch, and Scikit-learn, energizes developers with a comprehensive toolkit to execute and attempt diverse ML designs.
- Cross-Platform Compatibility
Python’s platform independence allows ML models to be perfectly released across various operating systems, making a smooth individual experience on numerous platforms.
- Easy Integration with Other Languages
Programmers can leverage Python’s ML abilities with libraries written in languages like C++ and Java, expanding the range of tools.
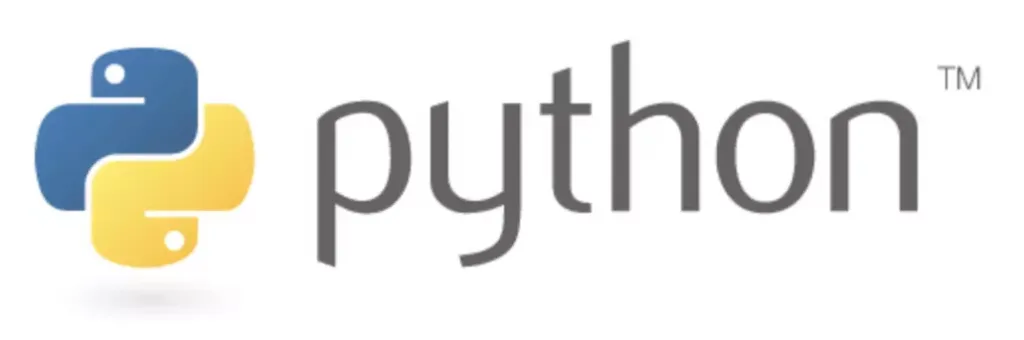
Machine Learning and AI: what’s the difference?
In the ever-evolving landscape of modern technology, 2 terms frequently come together and trigger curiosity – Artificial Intelligence (AI) and Machine Learning (ML). While both are indispensable components of contemporary technologies, it is important to understand their basic distinctions and the role Python plays in driving improvements in ML. This emphasizes the significant effect of Рython for machine learning in forming the mechanical scene.
1. Reactive Machines: The Foundation of AI
Reactive machines are the easiest type of AI, where systems respond based on predefined rules and patterns. These AI versions do not have memory or the ability to pick up from past experiences. Rather, they rely upon fixed algorithms to make decisions, making them suitable for particular, repetitive tasks. Reactive makers excel in efficiency and rate but require more versatility to deal with complicated, vibrant settings.
2. Theory of Mind: Understanding Human Cognition
The theory of mind delves into the world of understanding human emotions, beliefs, and purposes. While people have this remarkable cognitive capacity, AI systems are still beginning to develop such comprehension. In a legitimate theory of mind, AI would certainly be able to understand the frame of mind of others and predict their behavior based on these ideas. This degree of class in AI continues to be an ambitious and continuous location of study.
The joy of coding Python should be in seeing short, concise, readable classes that express a lot of action in a small amount of clear code — not in reams of trivial code that bores the reader to death.
Guido van Rossum
3. Limited Memory: Learning from Experience
AI frameworks with confined memory can choose from past encounters and alter their exercises as fundamental. These designs can alter to dynamic situations, making them fit for jobs with verifiable data. With each interaction and dataset, AI with restricted memory refines its decision-making strategy, steadily becoming more solid and exact.
4. Self-awareness: The Pinnacle of AI Advancement
At the crest of AI refinement exists self-awareness, a thought coordinating human awareness. Whereas individuals have self-awareness and an understanding of their state, capacities, and imperatives, duplicating this in AI frameworks remains a broad trouble. If an AI were to possess self-awareness, it would get its existence and environment, empowering it to form illuminated choices based upon a comprehensive understanding of its capacities.
Python’s versatility and expressive nature empower software engineers to make AI frameworks at changing degrees of refinement. From reactive devices to adaptations with restricted memory, Python serves as an ideal language for building savvy frameworks. Its clean express structure and impressive collections offer a supportive setting for AI analysts to investigate modern frontiers.
Python’s duty is a lot more obvious in the world of Artificial intelligence. Its simpleness and uniformity supply a user-friendly experience, drawing in developers from all backgrounds to embark on the ML trip. Python’s substantial community of ML libraries and structures, consisting of TensorFlow, PyTorch, and Scikit-learn, equips programmers to build detailed ML models with ease, thrusting the field of AI to brand-new heights.
Looking for a Python developer?
Contact UsWhy Python is Perfect for Machine Learning
Extensive Libraries and Frameworks
Python’s dominance in the machine learning realm is significantly attributed to its extensive array of libraries and frameworks. Some of the most popular include:
- TensorFlow: Developed by Google, TensorFlow is an open-source library that offers comprehensive tools for building and deploying machine learning models. Its flexibility and scalability make it ideal for both research and production environments.
- Keras: Now integrated into TensorFlow, Keras provides a user-friendly API for quickly building and experimenting with neural networks. Its simplicity allows for rapid prototyping, making it a favorite among developers and researchers.
- Scikit-learn: Known for its easy-to-use interface, Scikit-learn offers a wide range of algorithms for classification, regression, clustering, and more. It is highly regarded for its robust performance and ease of integration with other Python libraries.
- PyTorch: Developed by Facebook’s AI Research lab, PyTorch is praised for its dynamic computation graph and seamless integration with Python. It is widely used in academia and industry for developing deep learning models.
These libraries simplify the development process by providing pre-built functions and tools, allowing developers to focus on their specific problems rather than reinventing the wheel. The availability of these resources accelerates time-to-market, enabling businesses to implement machine learning solutions more quickly and efficiently.
Ease of Use and Readability
Python’s appeal in machine learning is partly due to its simple syntax and readability. The language is designed to be intuitive, making it accessible to both beginners and seasoned developers. Unlike other programming languages that may have complex syntax and steep learning curves, Python’s straightforward style allows for easier understanding and faster development.
When compared to languages like C++ or Java, which are also used in machine learning, Python’s learning curve is notably less steep. This ease of use not only accelerates the onboarding process for new developers but also facilitates better collaboration among teams. Python’s readability makes it easier to maintain and debug code, which is crucial for the iterative nature of machine learning projects.
Strong Community and Industry Support
One of Python’s greatest strengths is its vibrant community and strong industry backing. The Python community is extensive and highly active, providing a wealth of resources, including tutorials, forums, and documentation. This robust support network is invaluable for businesses, as it helps reduce development time and quickly resolves issues.
Industry giants like Google, Facebook, and Microsoft support Python, further cementing its status as a leading language for machine learning. This backing ensures continuous updates and improvements, keeping Python at the forefront of technological advancements. For businesses, this means access to cutting-edge tools and solutions, as well as a wealth of knowledge and expertise readily available for troubleshooting and development.
Integration Capabilities
Python’s ability to seamlessly integrate with other technologies and platforms is another reason it is ideal for machine learning in large enterprises. Its compatibility with various data science tools, cloud services, and big data platforms enhances its utility in complex business environments.
For instance, Python works well with data science tools like Jupyter Notebooks, which are widely used for data exploration and visualization. It also integrates effectively with cloud services such as AWS, Google Cloud, and Azure, enabling businesses to leverage scalable resources for their machine learning needs. Additionally, Python’s compatibility with big data platforms like Hadoop and Spark ensures that it can handle large volumes of data efficiently.
Case Studies of Python for Machine Learning Success
Python’s success in machine learning is exemplified by its widespread adoption across various industries. Here are some notable case studies of large enterprises leveraging Python for their machine learning initiatives:
- Google: As a major proponent of Python, Google uses it extensively for various machine learning projects. TensorFlow, a key library for machine learning, was developed by Google and is now used by the company for tasks ranging from search engine algorithms to artificial intelligence applications.
- Netflix: Netflix employs Python to power its recommendation engine, which suggests content to users based on their viewing history and preferences. The company uses machine learning algorithms implemented in Python to personalize recommendations and enhance user experience.
- Amazon: Amazon utilizes Python for its machine learning operations, particularly in its recommendation systems and AWS services. Python’s flexibility allows Amazon to build and deploy complex models that drive its product recommendations and customer insights.
- Uber: Uber relies on Python for a variety of machine learning applications, including route optimization and demand forecasting. The company uses Python to analyze vast amounts of data to improve its services and provide better experiences for users.
Python has made a significant impact
Python’s impact on various industries is particularly notable in finance, healthcare, and e-commerce:
- Finance: In the financial sector, Python is used for tasks such as risk assessment, fraud detection, and algorithmic trading. Its ability to handle complex calculations and analyze large datasets makes it a valuable tool for financial institutions seeking to enhance their decision-making processes and regulatory compliance.
- Healthcare: Python’s machine learning capabilities are transforming healthcare by enabling predictive analytics, personalized medicine, and medical imaging analysis. Companies are using Python to develop models that predict patient outcomes, identify potential health issues early, and improve overall treatment effectiveness.
- E-commerce: Python’s role in e-commerce is pivotal, with applications in recommendation systems, customer segmentation, and demand forecasting. By leveraging Python, e-commerce platforms can offer personalized shopping experiences, optimize inventory management, and drive sales growth.
Common Challenges in Machine Learning with Python
Common challenges in machine learning with Python include:
- Data Quality and Quantity: Insufficient or poor-quality data can hinder model performance.
- Feature Engineering: Selecting and crafting relevant features requires expertise.
- Model Overfitting/Underfitting: Balancing model complexity to avoid overfitting or underfitting.
- Computational Resources: High-performance models may require significant computational power.
- Hyperparameter Tuning: Finding the optimal settings for models can be time-consuming.
- Interpretability: Understanding and explaining model decisions can be challenging.
- Deployment and Integration: Moving from a development environment to production involves additional complexity.
Future-Proofing Your Business with Python
Machine learning is evolving rapidly, with several emerging trends shaping the future of the field:
- Advancements in AI and Deep Learning: Techniques such as reinforcement learning and generative adversarial networks (GANs) are driving innovations in AI. Python, with its robust libraries and frameworks, is well-positioned to support these advancements and remain at the cutting edge.
- Increased Focus on Ethical AI: As machine learning becomes more prevalent, there is a growing emphasis on developing ethical AI solutions. Python’s community-driven approach and extensive resources support efforts to create transparent and fair AI models.
- Integration with IoT and Edge Computing: Machine learning is increasingly being integrated with Internet of Things (IoT) devices and edge computing. Python’s versatility allows it to interface with these technologies, enabling real-time data processing and intelligent decision-making.
Final Thoughts
Python has proven to be an exceptional choice for machine learning, offering a powerful combination of extensive libraries, ease of use, strong community support, and seamless integration capabilities. By addressing common challenges and providing robust solutions, Python enables businesses to harness the full potential of machine learning. As the field continues to evolve, Python’s position at the forefront of innovation and its long-term benefits make it a strategic investment for future growth. Embracing Python for machine learning not only enhances operational efficiency but also positions businesses for continued success in a rapidly changing landscape.
At Ficus Technologies, we have witnessed firsthand the transformative power of Python in our projects. Our top-tier experts leverage Python’s simplicity and consistency to build innovative machine learning models that are reshaping industries. Python’s versatile libraries and frameworks have become the backbone of our AI-driven applications, allowing us to create intelligent systems that continuously adapt and learn from data.Looking ahead, Ficus Technologies is committed to staying at the cutting edge of machine learning advancements. With Python as our trusted ally, we continue to embrace innovation, shaping a world where intelligent algorithms redefine what is possible. For more information on how we can help you leverage Python for your machine learning needs, visit our contact page.
Python’s extensive community support is pivotal in nurturing the growth of its Machine Learning (ML) ecosystem. The dynamic community continually develops and shares new libraries, tools, and techniques, expanding the possibilities of ML. Active discussions, forums, and collaborative contributions create a rich knowledge pool for ML enthusiasts. This collective effort keeps the ecosystem vibrant and ensures that developers stay updated with the latest advancements. The collaborative environment fosters innovation, making Python a powerhouse for ML development with vast and ever-evolving resources.
Python seamlessly integrates with popular machine learning frameworks, making it a preferred language for developing sophisticated models. Major frameworks like TensorFlow, PyTorch, and Keras provide Python APIs, allowing developers to harness the power of these frameworks while leveraging Python’s simplicity and readability. This integration streamlines the machine learning workflow, enabling practitioners to access cutting-edge tools and technologies for tasks such as neural network design, training, and deployment. Python’s versatility and compatibility with these frameworks contribute to its dominance in the field of machine learning.
This article offers a clear and concise explanation for those curious about Python’s dominance in machine learning. It highlights Python’s advantages, such as its readability and the abundance of libraries and frameworks, making it a compelling choice for ML enthusiasts.