Welcome to the domain of data engineering, where the institutions of data engineering serve as the bedrock for overseeing and optimizing tremendous volumes of data. In this investigation, we’ll dig into the basics of data engineering, unraveling the essentials that support this basic space. Let’s set out on a travel to get it and ace the concepts typified by the term “data engineering explained”.
- Unraveling the core principles shaping data engineering, emphasizing its role as the foundation for managing vast datasets.
- Highlighting the critical components of data quality, scalability, speed, security, and integration, underscoring their importance in data engineering fundamentals.
- Detailing the multifaceted responsibilities of a data engineer, from designing robust systems to ensuring data quality and collaborating with cross-functional teams.
What is Data Engineering?
In the world of information management, data engineering stands as the foundation, encompassing the basics of data engineering that drive the whole process. At its core, data engineering is the art and scientific research of transforming raw information right into an organized and functional format. This entails a careful process that consists of collection, storage, and evaluation, ensuring that information is not simply a static entity but a dynamic force that powers decision-making.
The fundamentals of data engineering include constructing designs and systems capable of managing vast datasets. These datasets, usually originating from varied resources, require to be balanced and ready for downstream analytics. This fundamental understanding is vital for anyone browsing the facility landscape of information monitoring.
Data engineering belongs to building the infrastructure for a city– it entails mindful preparation, building and construction, and maintenance. By recognizing the details of data engineering, people gain insights right into just how information moves and is utilized within an organization. It’s the backstage pass to the world of analytics, scientific research, and decision-making.
Why Is Data Engineering Important?
The significance of data engineering cannot be exaggerated, and it pivots on a few key components that shape the bedrock of its importance.
- Data Quality
Making certain the high quality of information is a critical concern within data engineering. The fundamentals of data engineering need a careful technique to validate and improve information accuracy. This process is not just cleaning up messy datasets; it’s about developing a structure of trust in the information that organizations count on for essential decision-making. Data engineering discussed in this context stresses the importance of having a durable system in position to keep high data quality continually.
- Scalability
The importance of scalability in data engineering can not be overstated. As organizations expand, so does the quantity of data they create and procedures. The basics of data engineering dictate the design and execution of systems that can flawlessly scale to meet these boosting demands. Scalability is not just a function; it’s a requirement to make certain that the infrastructure can deal with the increasing range of details without jeopardizing performance.
- Speed
In the fast-paced world of data-driven decision-making, speed is an essential element. The fundamentals of data engineering focus on maximizing procedures to provide rapid results. From real-time analytics to speedy feedback mechanisms, the rate at which information is processed directly affects a company’s capacity to adapt and prosper in an affordable landscape. Data engineering explained highlights the demand for efficiency in processing to equal the dynamic nature of info.
- Security
Safety is an indispensable aspect of data engineering. Data engineering, clarified in the context of security, entails applying actions to guard sensitive details. This expands beyond fundamental encryption to extensive techniques that ensure conformity with data defense guidelines. An information breach can have serious consequences, making protection a non-negotiable part of the fundamentals of data engineering.
- Integration
The integration of varied information sources is a main task within data engineering. This includes harmonizing info from various databases, systems, and layouts into a cohesive structure. The fundamentals of data engineering stress the significance of seamless integration for a thorough view of the information landscape. It’s about breaking down silos and developing a combined system where info flows cohesively.
Person who is better at statistics than any software engineer and better at software engineering than any statistician.
Josh Wills
What Is The Role of a Data Engineer?
The role of a skilled data engineer is pivotal in the detailed dancing of information monitoring. At its significance, the data engineer is the designer behind the scenes, responsible for constructing and preserving the durable framework that allows the seamless circulation of information. Recognizing the basics of data engineering is basic for anyone stepping into this duty.
Basically, data engineering is a principal in the realm of data engineering. Their primary responsibility depends on translating the basics of data engineering right into sensible, workable steps. This includes creating and applying architectures that can manage large-scale data processing, ensuring the effective improvement of raw data into valuable insights.
Past the technical elements, a data engineer is a problem solver. They fix problems that might develop within the data framework, enhancing and making improvements in procedures to improve total performance. This vibrant function requires a deep understanding of the complexities of the data lifecycle, from acquisition to storage space and analysis.
The data engineering explained in the role of a data engineer extends to being a partner with cross-functional teams. Efficient communication is vital, as they need to comprehend the particular information demands of different divisions within a company. This joint method ensures that the information solutions executed align with the broader goals and objectives.
Furthermore, a data engineer is at the center of ensuring data quality. The basics of data engineering dictate that a data engineer should develop and enforce procedures that confirm and clean data, preserving its precision and dependability. This commitment to high-quality data is vital for promoting trust in the details utilized for decision-making.
Data Engineering Responsibilities
The responsibilities of a data engineer vary and include a large variety of jobs, all rooted in the core concepts of data engineering.
First and foremost, a data engineer is charged with establishing formulas and systems for reliable information handling. This entails recognizing the details of the information and designing remedies that cater to specific business requirements. The fundamentals of data engineering guide these endeavors, ensuring that the end result is not just a practical system but one that lines up with wider organizational purposes.
Protection is a critical duty within the role of a data engineer. Executing measures to secure delicate info is a consistent consideration. This entails not just guarding against outside risks but likewise making sure compliance with information protection regulations. The data engineering explained in this context highlights the important duty data engineers play in maintaining the stability and confidentiality of organizational data.
Furthermore, a data engineer takes part in optimizing database performance. This entails fine-tuning inquiries, indexing information for efficient access, and guaranteeing that the data source framework can manage the needs positioned upon it. Scalability, a vital idea in the basics of data engineering, is a recurring theme in these optimization efforts.
Collaboration is the foundation of a data engineer’s duties. Functioning very closely with information researchers, analysts, and various other stakeholders, they guarantee that information remedies straighten with the overall approach of the company. This joint spirit extends to fixing issues that may arise, calling for a positive and problem-solving state of mind.
Are you interested in how to optimize your data infrastructure?
Contact UsData Engineering Trends
In the ever-evolving landscape of data engineering, remaining abreast of emerging trends is critical. Two noteworthy patterns forming the future of data engineering consist of:
AI-driven Development
In the ever-evolving landscape of data engineering, one famous pattern is the integration of artificial intelligence (AI) right into development procedures, an indication of the consistent advancement of the basics of data engineering. Data engineering discussed within the context of AI-driven growth represents a standard change. It entails leveraging machine learning formulas and advanced analytics devices to automate and boost different aspects of data engineering operations.
AI-driven growth in data engineering brings forth a brand-new age of effectiveness and advancement. Data engineering now harnesses the power of intelligent algorithms to automate recurring jobs, optimizing data processing pipelines. This not only accelerates the speed at which data is dealt with but also decreases the margin for error, lining up with the overarching basics of data engineering.
Moreover, the integration of AI in data engineering contributes to smarter decision-making. The formulas, constantly learning from data patterns, offer predictive insights, shaping a proactive strategy to analytical. This transformative trend not only redefines the conventional limits of data engineering but also emphasizes the need for experts to stay abreast of these improvements, stressing the continuous need to be fluent in the fundamentals of data engineering.
The synergy between AI and data engineering is not simply a technical marriage; it stands for a basic shift in how data is refined and made use of. Welcoming AI-driven advancement in data engineering isn’t simply an alternative– it’s becoming an imperative for companies seeking a competitive edge in the data-driven era.
Software Development
An additional noteworthy trend reshaping the landscape of data engineering is the boosting partnership between data engineering and software program development, further obscuring the lines between both realms. Comprehending the basics of data engineering currently prolongs beyond typical data handling approaches to consist of smooth integration with software development practices. Data engineering, explained in this context, highlights the convergence of these self-controls to develop a holistic technique for information monitoring.
The symbiosis between data engineering and software application advancement generates a myriad of benefits. It causes the creation of durable devices, platforms, and applications that streamline information processes, aligning perfectly with the basics of data engineering. This cooperation makes sure that information options are not only practically sound but also straightforward, accommodating a wider audience within an organization.
Moreover, this trend emphasizes dexterity and adaptability. The integration of software application development practices within data engineering allows for more adaptable and responsive options. Modifications in data demands can be quickly fit, echoing the concepts of scalability inherent in the basics of data engineering.
As software program development comes to be an essential part of the data engineering landscape, experts in both domains find themselves working hand in hand. This cooperation leads to a convergence of abilities, where data engineers come to be proficient at coding, and software application developers gain insights right into the ins and outs of data architecture. The result is a powerful harmony that improves just how companies come close to data-driven initiatives, enveloping the significance of data engineering explained in the dynamic interaction between these two trends.
Data Engineering Tools
Data engineering tools play a key role in the fundamentals of data engineering, providing the foundation for managing, processing and optimizing massive amounts of data. These tools embody the fundamentals of data engineering, providing the infrastructure and efficiency needed to successfully work with data.
1. Amazon Redshift
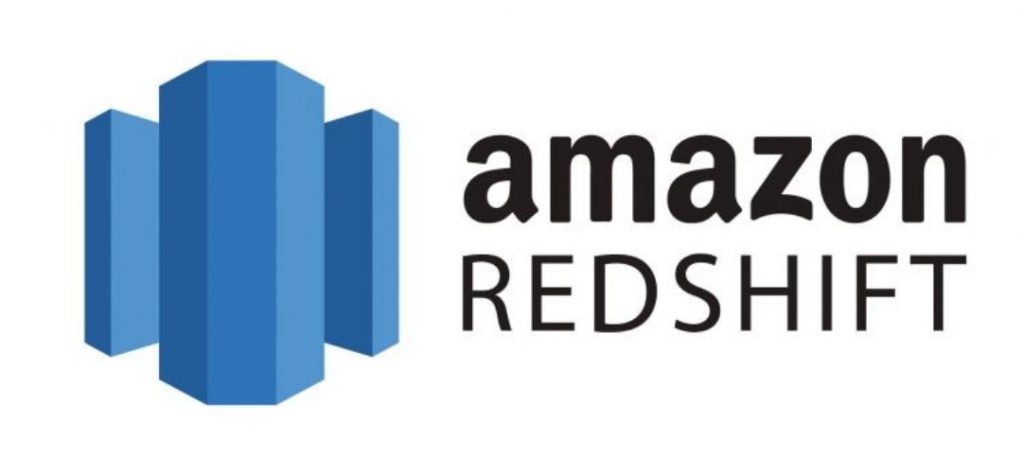
Amazon Redshift, a foundational tool in data engineering, is fully managed cloud storage from Amazon. As the foundation of data engineering, it simplifies data warehouse customization. As a powerful tool for thousands, it embodies the fundamentals of data engineering while providing easy scalability as your business grows. In essence, Amazon Redshift is data engineering at its most efficient and practical level.
2. Big Query
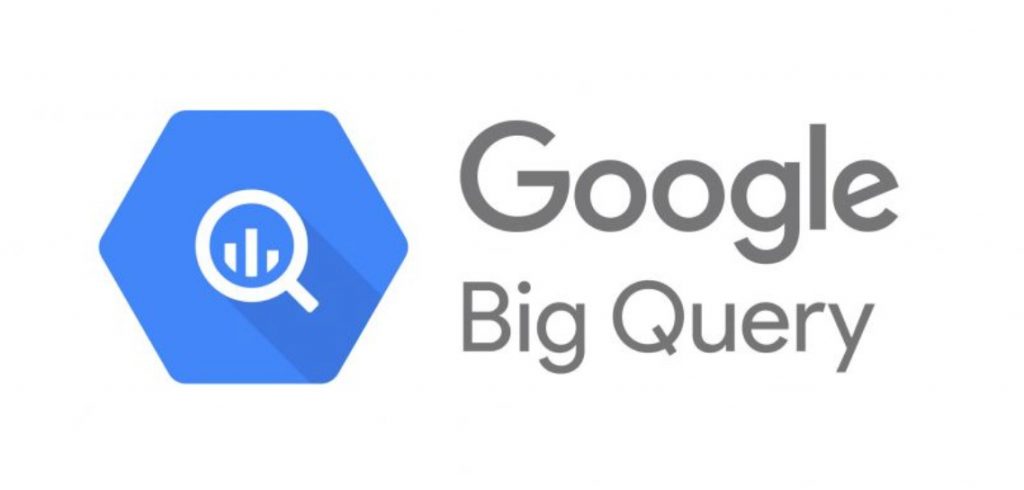
BigQuery, a key component of data engineering, is a fully managed cloud data warehouse on the Google Cloud Platform that demonstrates the fundamentals of data engineering. Ideal for scaling, analysts and engineers integrate it seamlessly as their data grows, showcasing the fundamentals of data engineering explained through practical application.
3. Tableau
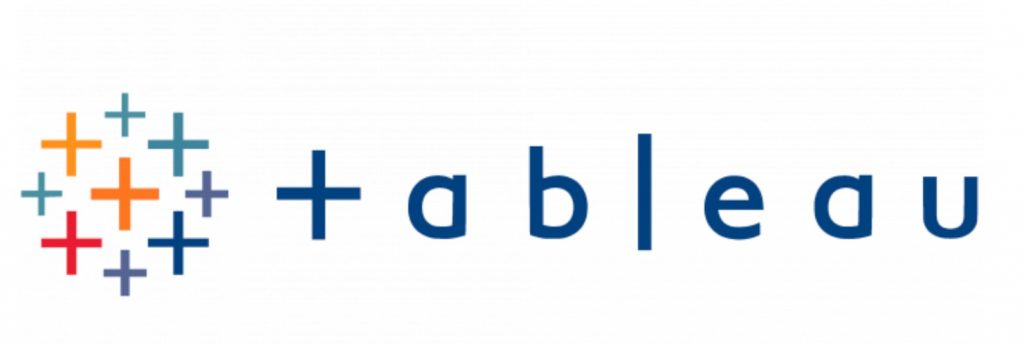
Tableau, the cornerstone of data engineering, ranks second among the most used BI tools. As one of the oldest data visualization solutions, it does a great job of collecting and extracting data from a variety of sources. With its user-friendly drag-and-drop interface, Tableau embodies the fundamentals of data engineering, facilitating seamless collaboration between departments. Data engineering is explained through intuitive dashboards created by experienced managers.
4. Looker
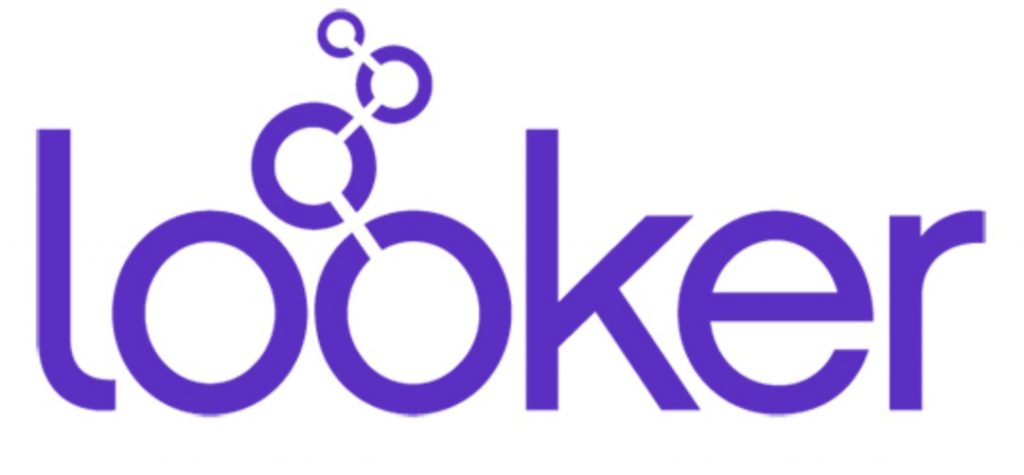
Looker, an innovator in data engineering, is revolutionizing BI software by empowering employees to visualize data. Gaining widespread adoption, especially among engineering teams, it goes beyond traditional BI tools with its original LookML layer, a language that defines dimensions, aggregates, and relationships of data in SQL databases. A recent addition, Spectacles, simplifies the management of the LookML layer, allowing data engineers to increase accessibility to non-technical users by explaining the basics of data engineering.
5. Apache Spark
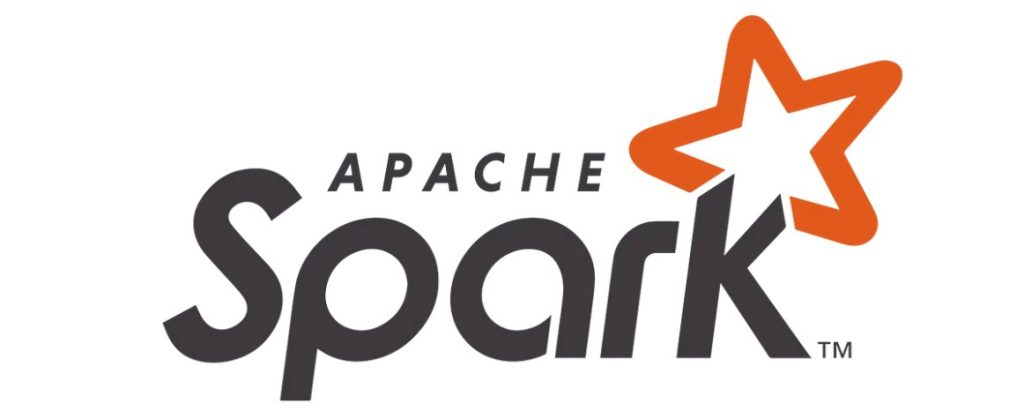
Apache Spark, a key force in data engineering, is a unified, open-source analytics engine designed for large-scale data processing. As a robust data processing engine, it quickly handles the task of processing vast data sets by distributing them across multiple computers. These qualities are fundamental to big data and machine learning, epitomizing the fundamentals of data engineering explained through unprecedented computational power.
Final thought
Finally, diving right into the principles of data engineering exposes its essential function in handling and enhancing vast volumes of data. From making certain data quality and scalability to highlighting speed, safety, security, and combination, the basics highlight the essential value of this area. A data engineer’s role as the engineer behind details flow, issue solver, and collaborator highlights the vibrant nature of their obligations. Emerging trends like AI-driven advancement and the expanding harmony with software program development further shape the landscape. Browsing the range of data engineering tools is crucial, with data sources, combination systems, refining structures, and visualization devices playing key functions.
In this progressing field, Ficus Technologies stands out as an overview and companion. With knowledge in data engineering fundamentals, critical application, facilities optimization, and proficiency of devices, Ficus Technologies supplies a transformative journey. As a joint force dedicated to high quality and security, Ficus Technologies guarantees organizations stay at the leading edge of technology trends.
Data engineers uphold data quality and integrity through meticulous processes. They implement robust data validation protocols to identify anomalies and discrepancies, ensuring accuracy. Employing data cleaning techniques, such as handling missing values and outliers, further enhances data reliability. Additionally, data engineers establish data governance frameworks, enforcing standardized formats and definitions. Continuous monitoring and auditing of data pipelines are integral, enabling prompt identification and rectification of any issues. By adhering to best practices, maintaining comprehensive documentation, and leveraging automated tools, data engineers safeguard the quality and integrity of the data throughout its lifecycle.
Data engineering is pivotal in the realm of machine learning and AI by providing the foundational infrastructure for robust data pipelines. It involves collecting, cleaning, and transforming data into a format suitable for model training. Data engineers create environments conducive to algorithm development, ensuring seamless integration of data into machine learning models. The efficiency of these processes directly influences the performance and accuracy of AI applications. Data engineering establishes the groundwork for extracting meaningful insights, enabling machine learning algorithms to learn and adapt, ultimately contributing to the advancement and effectiveness of artificial intelligence across various domains.